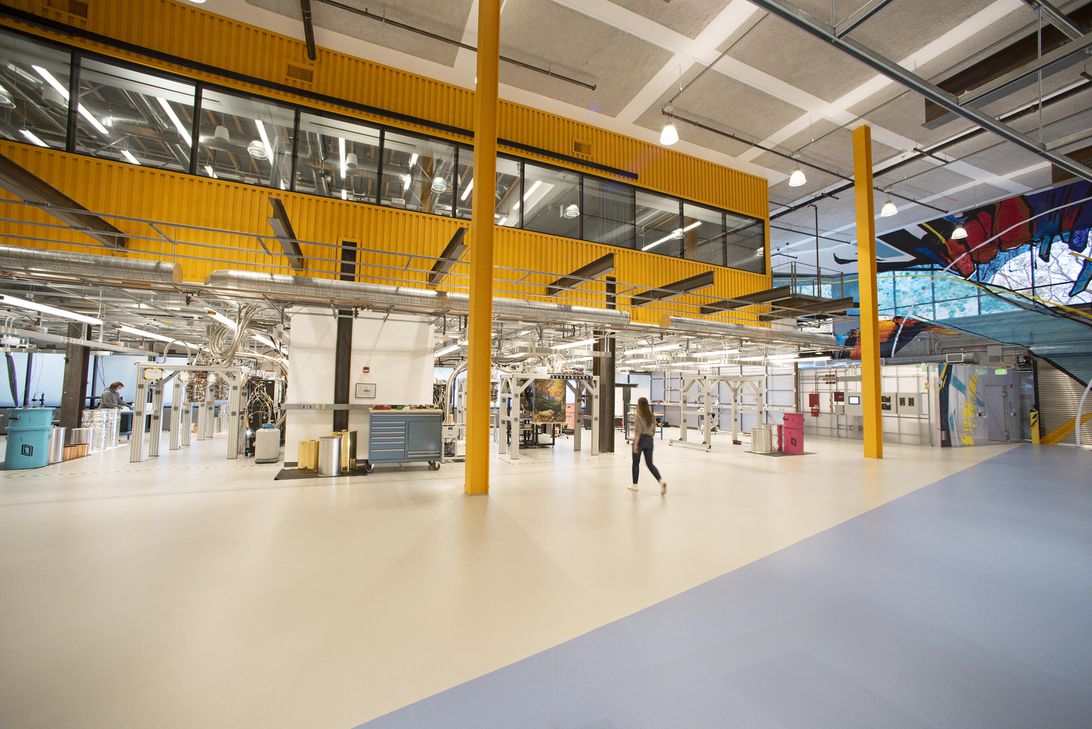
Google’s new Quantum AI Campus in Santa Barbara, California, will employ hundreds of researchers, engineers and other staff.
Stephen Shankland/CNET
Google has begun building a new and larger quantum computing research center that will employ hundreds of people to design and build a broadly useful quantum computer by 2029. It’s the latest sign that the competition to turn these radical new machines into practical tools is growing more intense as established players like IBM and Honeywell vie with quantum computing startups.
The new Google Quantum AI campus is in Santa Barbara, California, where Google’s first quantum computing lab already employs dozens of researchers and engineers, Google said at its annual I/O developer conference on Tuesday. A few initial researchers already are working there.
One top job at Google’s new quantum computing center is making the fundamental data processing elements, called qubits, more reliable, said Jeff Dean, senior vice president of Google Research and Health, who helped build some of Google’s most important technologies like search, advertising and AI. Qubits are easily perturbed by outside forces that derail calculations, but error correction technology will let quantum computers work longer so they become more useful.
“We are hoping the timeline will be that in the next year or two we’ll be able to have a demonstration of an error-correcting qubit,” Dean told CNET in a briefing before the conference.
Quantum computing is a promising field that can bring great power to bear on complex problems, like developing new drugs or materials, that bog down classical machines. Quantum computers, however, rely on the weird physical laws that govern ultrasmall particles and that open up entirely new processing algorithms. Although several tech giants and startups are pursuing quantum computers, their efforts for now remain expensive research projects that haven’t proven their potential.
“We hope to one day create an error-corrected quantum computer,” said Sundar Pichai, chief executive of Google parent company Alphabet, during the Google I/O keynote speech.
Error correction combines many real-world qubits into a single working virtual qubit, called a logical qubit. With Google’s approach, it’ll take about 1,000 physical qubits to make a single logical qubit that can keep track of its data. Then Google expects to need 1,000 logical qubits to get real computing work done. A million physical qubits is a long way from Google’s current quantum computers, which have just dozens.
One priority for the new center is bringing more quantum computer manufacturing work under Google’s control, which, when combined with an increase in the number of quantum computers, should accelerate progress.
Google is spotlighting its quantum computing work at Google I/O, a conference geared chiefly for programmers who need to work with the search giant’s Android phone software, Chrome web browser and other projects. The conference provides Google a chance to show off globe-scale infrastructure, burnish its reputation for innovation and generally geek out. Google is also using the show to tout new AI technology that brings computers a bit closer to human intelligence and to provide details of its custom hardware for accelerating AI.
As one of Google’s top engineers, Dean is a major force in the computing industry, a rare example of a programmer to be profiled in The New Yorker magazine. He’s been instrumental in building key technologies like MapReduce, which helped propel Google to the top of the search engine business, and TensorFlow, which powers its extensive use of artificial intelligence technology. He’s now facing cultural and political challenges, too, most notably the very public departure of AI researcher Timnit Gebru.
Google’s TPU AI accelerators
At I/O, Dean also revealed new details of Google’s AI acceleration hardware, custom processors it calls tensor processing units. Dean described how the company hooks 4,096 of its fourth-generation TPUs into a single pod that’s 10 more powerful than earlier pods with TPU v3 chips.
“A single pod is an incredibly large amount of computational power,” Dean said. “We have many of them deployed now in many different data centers, and by the end of the year we expect to have dozens of them deployed.” Google uses the TPU pods chiefly for training AI, the computationally intense process that generates the AI models that later show up in our phones, smart speakers and other devices.
Previous AI pod designs had a dedicated collection of TPUs, but with TPU v4, Google connects them with fast fiber-optic lines so different modules can be yoked together into a group. That means modules that are down for maintenance can easily be sidestepped, Dean said.
Google’s TPU v4 pods are for its own use now, but they’ll be available to the company’s cloud computing customers later this year, Pichai said.
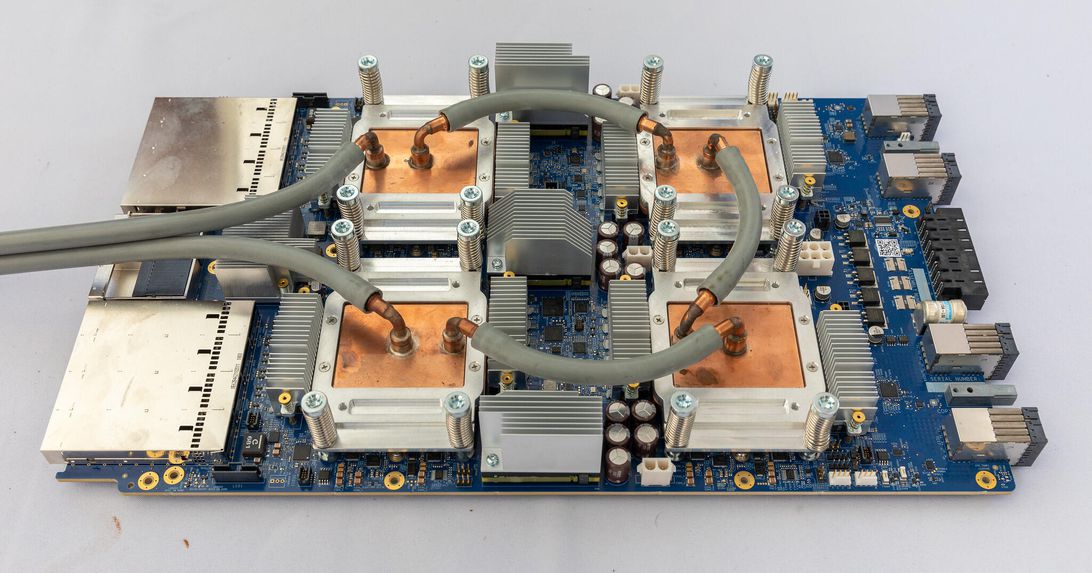
Google’s tensor processing unit processors, used to accelerate AI work, are liquid-cooled. These are third-generation TPU processors.
Stephen Shankland/CNET
The approach has been profoundly important to Google’s success. While some computer users focused on expensive, ultra-reliable computing equipment, Google has employed cheaper equipment since its earliest days. However, it designed its infrastructure so that it could continue working even when individual elements failed.
Google is also trying to improve its AI software with a technique called multitask unified model, or MUM. Today, separate AI systems are trained to recognize text, speech, photos and videos. Google wants a broader AI that spans all those inputs. Such a system would, for example, recognize a leopard regardless of whether it saw a photo or heard someone speak the word, Dean said.